data model
A data model is an abstract view over the data that hides the way it is stored physically.
The JSON Information Set
The appropriate abstraction for any JSON document is a tree. The nodes of that tree, which are JSON logical values, are naturally of six possible kinds: the six syntactic building blocks of JSON.
These are the four leaves corresponding to atomic values:Strings, Numbers,Booleans, Nulls. As well as two intermediate nodes: Objects, Arrays. These nodes are generally called information items and form the logical building blocks of the model, called information set.
The XML Information Set
A fundamental difference between JSON trees and XML trees is that for JSON, the labels (object keys) are on the edges connecting an object information item to each one of its children information items. In XML, the labels (these would be element and attribute names) are on the nodes (information items) directly.
In XML, there are many more information items: Document information items, Element, Attribute, Character, Comment, Processing instruction, Name space, Unexpanded entity reference, DTD, Unparsed entity, Notation. Here we only focus on documents, elements, attributes, and characters.
Validation
Once documents, JSON or XML, have been parsed and logically abstracted as a tree in memory, the natural next step is to check for further structural constraints.
In a relational database, the schema of a table is defined before any data is populated into the table. Thus, the data in the table is guaranteed, at all times, to fulfil all the constraints of the schema. The schema was enforced at write time (schema on write). A collection of JSON and XML documents out there can exist without any schema and contain arbitrary structures. Validation happens “ex post,” that is, only after reading the data (schema on read).
JSON and XML documents undergo two steps: a well-formedness check: attempt to parse the document and construct a tree representation in memory;(if the first step succeeded) a validation check given a specific schema. Note that, unlike well-formedness, validation is schema dependent: a given well-formed document can be valid against schema A and invalid against schema B.
Item types
A fundamental aspect of validation is the type system. A well-designed type system, in turn, allows for storing the data in much more efficient, binary formats tailored to the model.
Atomic types
Atomic types correspond to the leaf of a tree data model: these are types that do not contain any further nestedness. The kinds of atomic types available are also relatively standard and common to most technologies. Also, all atomic types have in common that they have a logical value space and a lexical value space.
An atomic type also has a (not necessarily injective) mapping from its lexical value space to its logical value space (e.g., mapping the hexadecimal literal x10 to the mathematical integer sixteen).
Atomic types can be in a subtype relationship: a type is a subtype of another type if its logical value space is a subset of the latter.
Strings
In “pure computer science” textbooks, strings are often presented as structured values rather than as atomic values because of their complexity on the physical layer. However, for us data scientists, strings are atomic values.
Numbers: integers
Integers correspond to finite cardinalities (counting) as well as their negative counterparts. In older programming languages, support for integers used to be bounded. However, in modern databases, it is customary to support unbounded integers. Engines can optimize computations for small integers, but might become less efficient with very large integers.
Numbers: decimals
Decimals correspond to real numbers that can be written as a finite sequence of digits in base 10, with an optional decimal period.
Numbers: floating-point
Support for the entire decimal value space can be costly in performance. In order to address this issue, a floating-point standard (IEEE 754) was invented and is still very popular today.
Floating-point numbers are limited both in precision and magnitude (both upper and lower) in order to fit on 32 bits (float) or 64 bits (double). Floats have about 7 digits of precision and their absolute value can be between roughly 10^−37 and 10^37, while doubles have 15 digits of precision and their absolute value can be between roughly 10^−307 and 10^308.
Booleans
The logical value space for the Boolean type is made of two values: true and false as in NoSQL queries, two-valued logic is typically assumed.
Dates and times
Dates are commonly using the Gregorian calendar (with some technologies possibly supporting more) with a year (BC or AD), a month and a day of the month. Times are expressed in the hexagesimal (60) basis with hours, minutes, seconds, where the seconds commonly go all the way to microseconds (six digits after the decimal period). Datetimes are expressed with a year, a month, a day of the month, hours, minutes and (decimal) seconds.
Timestamp values are typically stored as longs (64-bit integers) expressing the number of milliseconds elapsed since January 1, 1970 by convention.
XML Schema, JSound and JSONiq follow the ISO 8601 standard, where lexical values look like so (with many parts optional): 2022-08-07T14:18:00.123456+02:00.
Durations
The lexical representation can vary, but there is a standard defined by ISO 8601 as well, starting with a P and prefixing sub-day parts with a T.
4 days, 3 hours, 2 minutes and 1.123456 seconds: P4DT3H2M1.123456S.
Binary data
Binary data is, logically, simply a sequence of bytes. There are two main lexical representations used in data: hexadecimal and base64. Hexadecimal expresses the data with two hexadecimal digits per byte. Base 64, formally, does the same but in the base 64, which “wastes” less lexical space in the text. It does so by encoding the bits six by six, encoding each sequence of six bits with one base-64 digit.
Null
A schema can either allow, or disallow the null value.
XML also supports null values, but calls them “nil” and does so with a special attribute and no content rather than with a lexical representation
Structured types
Lists
Lists correspond to JSON arrays and are ordered sequences of (atomic or structured) values.
Records
Records, or structs, correspond to JSON objects and are maps from strings to values.
Maps
Maps (not be confused with records, which are similar) are maps from any atomic value to any value, i.e., generalize objects to keys that are not necessarily strings (e.g., numbers, dates, etc).
With a schema, it is possible to restrict the type of the keys, as well as the type of the values. However, unlike records, the type of the values must be the same for all keys.
Sets
Sets are like lists, but without any specific ordering, and without duplicate values.
XML elements and attributes
XML Schema stands apart from most other technologies and formats, in that it does not offer specific support for records and maps; it offers some limited support for lists, but considers them to be simple types, which are “inbetween” atomic types and structured types. n XML Schema, structure is obtained, instead, with elements and attributes, and the machinery for elements and attributes is highly specific to XML.
Type names
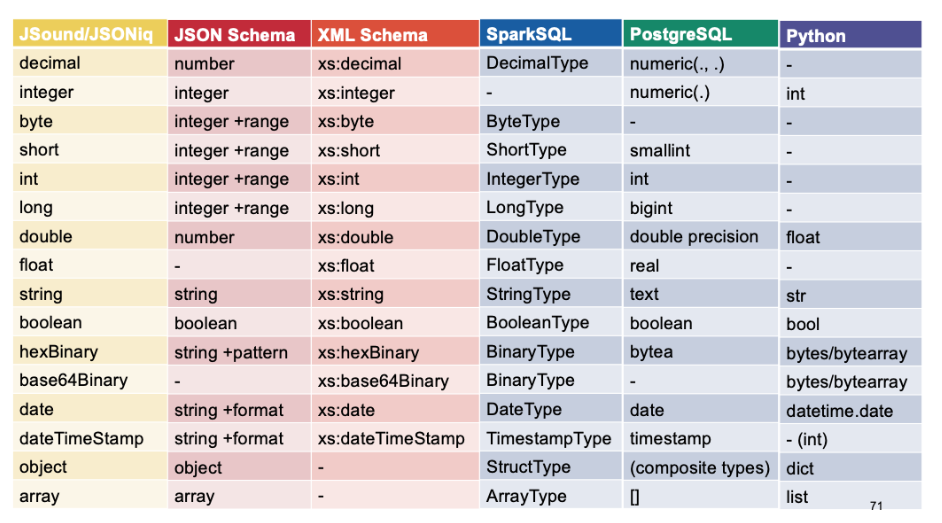
Sequence types
Cardinality
Many type system give options regarding the number of occurrences of items in a sequence.
Collections vs. nested lists
A collection of items is on the outer level, and can be massively large (billions, trillions of items).
A list (or array) of items, however, usually refers to a nested structure, for example an array nested inside a document or object. Such lists of items are usually restricted in size for reasons of performance and scalability.
It is thus important to keep this subtle difference in mind, in particular, do not confuse a collection of integers with a collection that contains a single array of integers.
JSON validation
Validating flat objects
JSound is a schema language that was designed to be simple for 80% of the cases, making it particularly suitable in a teaching environment.It is independent of any programming language.JSON Schema is another technology for validating JSON documents. The available JSON Schema types are string, number, integer, boolean, null, array and object.
An example for a json document is like:
{ “name” : “Einstein”, “first” : “Albert”, “age” : 142 }
The JSound and the JSON Schema are as follows:
{ “name” : “string”, “first” : “string”, “age” : “integer” }
{ “type” : “object”, “properties” : { “name” : “string”, “first” : “string”, “age” : “number” } }.
The type system of JSON Schema is thus less rich than that of JSound, but extra checks can be done with so-called formats, which include date, time, duration, email, and so on including generic regular expressions.
Requiring the presence of a key
It is possible to require the presence of a key by adding an exclamation mark in JSound. The equivalent JSON Schema uses a “required” property associated with the list of required keys to express the same.
Open and closed object types
In the JSound compact syntax, extra keys are forbidden. The schema is said to be closed. There are ways to define JSound schemas to allow arbitrary additional keys (open schemas), with a more verbose syntax. Unlike JSound, in JSON Schema, extra properties are allowed by default. JSON Schema then allows to forbid extra properties with the “additionalProperties” property.
Nested structures
{ “numbers” : [ “integer” ] }
Every schema can be given a name, turning into a type.
JSound allows for the definition not only of arbitrary array and object types, but also user-defined types.
Primary key constraints, allowing for null, default values
There are a few more features available in the compact JSound syntax (not in JSON Schema) with the special characters @, ? and =. The question mark (?) allows for null values. The arobase (@) indicates that one or more fields are primary keys for a list of objects that are members of the same array. The equal sign (=) is used to indicate a default value that is automatically populated if the value is absent.
Note that validation only checks whether lexical values are part of the type’s lexical space.
Accepting any values
Accepting any values in JSound can be done with the type “item”, which contains all possible values. In JSON Schema, in order to declare a field to accept any values, you can use either true or an empty object in lieu of the type. JSON Schema additionally allows to use false to forbid a field.
Type unions
In JSON Schema, it is also possible to combine validation checks with Boolean combinations. JSound schema allows defining unions of types with the vertical bar inside type strings, like so: “string|array”.
Type conjunction, exclusive or, negation
In JSON Schema only (not in JSound), it is also possible to do a conjunction (logical and), as well as exclusive or (xor), as well as negation.
XML validation
Simple types
All elements in an XML Schema are in a namespace, the XML Schema namespace. It is recommended to stick to the prefix xs, or xsd, which is also quite popular. We do not recommend declaring the XML Schema namespace as a default namespace, because it can create confusion in several respects.
The list of predefined atomic types is the same as in JSound, except that in XML Schema, all these predefined types live in the XML Schema namespace and thus bear the prefix xs as well.
Builtin types
XML Schema allows you to define user-defined atomic types, for example restricting the length of a string to 3 for airport codes, and then use it with an element.
Complex types
It is also possible to constrain structures and the element/attribute/text hierarchy with complex types applying to element nodes.
There are four main kinds of complex types:• complex content: there can be nested elements, but there can be no text nodes as direct children. • simple content: there are no nested elements: just text, but attributes are also possible. • empty content: there are neither nested elements nor text, but attributes are also possible. • mixed content: there can be nested elements and it can be intermixed with text as well.
Attribute declarations
Finally, all types of content can additionally contain attributes. Attributes always have a simple type.
Anonymous types
Finally, it is not mandatory to give a name to all types. Be careful: if there is neither a type attribute nor a nested type declaration, then anything is allowed!
Miscellaneous
Finally, XML Schema documents are themselves XML documents, and can thus be validated against a “schema or schemas”, itself written as an XML Schema.This schema has the wonderful property of being valid against itself.
Data frames
Heterogeneous, nested datasets
The beauty of the JSON data model is that, unlike the relational model and the CSV syntax, it supports nested, heterogeneous datasets, while also supporting as a particular case flat, homogeneous datasets.
Dataframe visuals
There is a particular subclass of semi-structured datasets that are very interesting: valid datasets, which are collections of JSON objects valid against a common schema, with some requirements on the considered schemas. The datasets belonging to this particular subclass are called data frames, or dataframes.
Specifically, for the dataset to qualify as a data frame, firstly, we forbid schemas that allow for open object types. secondly, we forbid schemas that allow for object or array values to be too permissive and allow any values. We, however, include schemas that allow for null values and/or absent values. Relational tables are data frames, while data frames are not necessarily relational tables. Data frames are a generalization of (normalized) relational tables allowing for (organized and structured) nestedness.
exercies
complextType cannot contain character by default but with mixed=”true” it can.
protobuf
convert json-like data to columnar representation(why we want this: make it more efficient to get relevant data rather than get the whole table).
convert the columnar representation back to the original format. Replace the missing field with NULL. It’s a “lossless” conversion.
Dremel
optional: 0 or 1. repeated: 1 or more.