data cubes
slides
OLTP VS OLAP:
record keeping vs decision support
read-intensive vs write-intensive
detailed individual records vs summarized data
Lots of transactions on small portions of data vs Large portions
of the data.
fully interactive vs slow interactive.
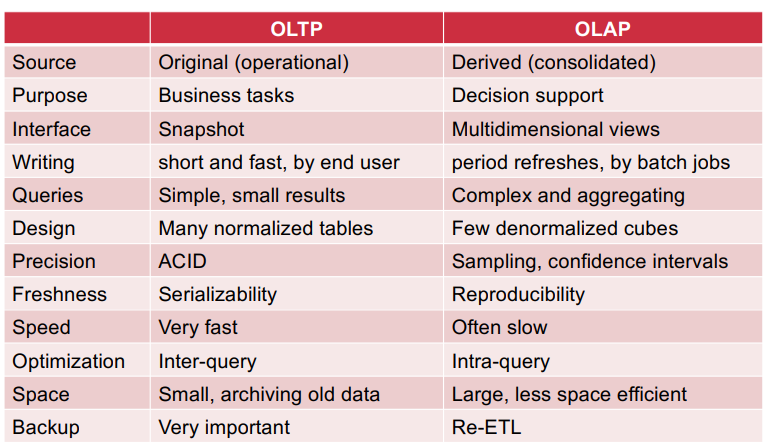
ETL: Extract Transform Load
slicing and dicing,Pivoting
Slicing involves selecting a specific “slice” or subset of the data cube by fixing one or more dimensions at a particular value. Dicing involves creating a subcube by selecting specific values for two or more dimensions. It’s like slicing, but you are selecting a rectangular subset of the cube, cutting through more than one dimension. Pivoting is another operation often used alongside slicing and dicing. It involves rotating the data cube to view it from a different perspective by swapping the positions of dimensions.
Aggregation and roll-up
These operations allow users to summarize and view data at different levels of granularity within a multidimensional dataset. Roll-up is a specific form of aggregation that involves moving from a lower level of detail to a higher level by collapsing one or more dimensions(moving up the hierarchy from a finer level of granularity (monthly) to a coarser level (quarterly)).
Two flavors of OLAP: ROLAP and MOLAP
ROLAP (Relational Online Analytical Processing) and MOLAP (Multidimensional Online Analytical Processing) are two different approaches to organizing and processing data in the context of Online Analytical Processing (OLAP) systems. In summary, the main difference between ROLAP and MOLAP lies in how they store and organize data. ROLAP uses relational databases to store multidimensional data in tables, while MOLAP uses specialized databases with a cube structure optimized for efficient multidimensional analysis.
fact table and Satellite table
Fact tables are surrounded by dimension tables in a star schema. They usually have foreign key relationships with dimension tables, linking to various dimensions that provide context to the measures. Satellite tables are used to store additional details about dimension members that are not part of the core structure of the dimension table. Satellite tables typically have a foreign key relationship with the main dimension table, linking to the primary key of the dimension. They can be joined to the main dimension table to enrich the analysis with additional context.
star schema and snow-flake schema
In a star schema, there is a central fact table surrounded by dimension tables. The fact table contains quantitative data (measures), and the dimension tables store descriptive data related to the measures. A snowflake schema is an extension of the star schema, where dimension tables are normalized into multiple related tables. This normalization leads to a structure resembling a snowflake when visualized, as opposed to the star-like structure of a star schema.
SQL querying tables and MDX querying Cubes
MDX stands for Multi-Dimensional
eXpressions.
“Roll-up” and “drill down”
Roll-up involves summarizing data at a higher level of aggregation or moving from a lower level of detail to a higher level. It’s a way of viewing data at a coarser level in the hierarchy. Drill down is the opposite of roll-up. It involves accessing more detailed information or moving from a higher level of aggregation to a lower, more detailed level.
GROUPING SETS, CUBE, ROLLUP
GROUPING SETS is a SQL feature that allows you to specify multiple grouping sets within a single query. The CUBE operation is an extension of GROUP BY that generates all possible combinations of grouped elements. ROLLUP is another extension of GROUP BY that generates subtotals and grand totals for a specified set of columns. It creates a hierarchy of grouping levels, calculating subtotals as it rolls up the hierarchy. Note that the column order in rollup is important.
book
exercise
concepts of fact tables;
pivot table;
SQL query with grouping set, cube…
Graph Databases
sildes
Index-free adjacency
With index-free adjacency, graph databases are designed in such a way that relationships between nodes are directly accessible without the need for an index structure. Instead of traversing indexes to find related nodes, the relationships are stored alongside the nodes, allowing for faster and more efficient traversal.Each node maintains direct pointers or references to its neighboring nodes, making it possible to navigate from one node to another without the need for an explicit index lookup. This design principle is particularly beneficial for scenarios where traversing relationships in a graph is a common and performance-critical operation.
Property Graph and Triple stores (RDF)
Labeled property graphs: ingredients: Nodes, Edges,Properties,Labels.
A property graph is a graph data model that consists of nodes, edges, and properties.RDF is a standard for representing data about resources in the form of subject-predicate-object triples.
Cypher
Cypher is a query language commonly used with property graph databases like Neo4j.
SPARQL
SPARQL is a query language commonly used with RDF triple stores.
Neo4j
Data replication;
sharding:neo4j fabric;
Caching and pages;
RDF
IRI (=URI for all practical purposes);
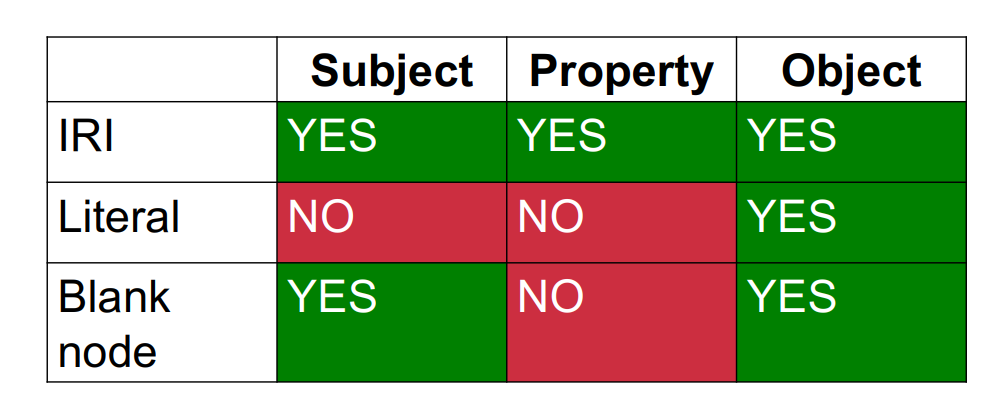
RDF formats:RDF/XML;Turtle;JSON-LD;RDFa;N-Triples.
book
Labeled property graph model vs. triple stores
labeled property graphs enhance mathematical graphs with extra ingredients: properties, and labels.
Labels are some sort of “tags”, in the form of a string, that can be attached to a node or an edge.
Each node and each edge can be associated with a map from strings to values, which represents its properties.
Triple stores are a different and simpler model. It views the graph as nodes and edges that all have labels, but without any properties.
The graph is then represented as a list of edges, where each edge is a triple with the label of the origin node (called the subject), the label of the edge (called the property), and the label of the destination node (called the object).
Labels can be:URIs,Literals, that is, atomic values,Literals are only allowed as objects. Absent, in which case the node is called a blank node. Blank nodes are only allowed as subjects or objects, but not as properties.
cypher
Other graph databases have other means of querying data. Many, including Neo4j, support the RDF query language SPARQL and the imperative, path-based query language Gremlin.
Cypher enables a user (or an application acting on behalf of a user) to ask the database to find data that matches a specific pattern. Colloquially, we ask the database to “find things like this.” And the way we describe what “things like this” look like is to draw them, using ASCII art.
The MATCH clause is at the heart of most Cypher queries.
native graph processing
To understand why native graph processing is so much more efficient than graphs based on heavy indexing, consider the following. Depending on the implementation, index lookups could be O(log n) in algorithmic complexity versus O(1) for looking up immediate relationships. To traverse a network of m steps, the cost of the indexed approach, at O(m log n), dwarfs the cost of O(m) for an implementation that uses index-free adjacency.
With index-free adjacency, bidirectional joins are effectively precomputed and stored in the database as relationships
stores
Neo4j stores graph data in a number of different store files. Each store file contains the data for a specific part of the graph (e.g., there are separate stores for nodes, relationships, labels, and properties). Like most of the Neo4j store files, the node store is a fixed-size record store, where each record is nine bytes in length. Fixed-size records enable fast lookups for nodes in the store file.
CYPHER,Traverser API and Core API
Neo4j’s Core API is an imperative Java API that exposes the graph primitives of nodes, relationships, properties, and labels to the user. When used for reads, the API is lazily evaluated, meaning that relationships are only traversed as and when the calling code demands the next node.
The Traversal Framework is a declarative Java API. It enables the user to specify a set of constraints that limit the parts of the graph the traversal is allowed to visit.
Cypher can be more tolerant of structural changes—things such as variable-length paths help mitigate variation and change.
however, the Traversal Framework tends to perform marginally less well than a well-written Core API query.
Choosing between the Core API and the Traversal Framework is a matter of deciding whether the higher abstraction/lower coupling of the Traversal Framework is sufficient, or whether the close-to-the-metal/higher coupling of the Core API is in fact necessary for implementing an algorithm correctly and in accordance with our performance requirements.
exercise
Querying trees
slides
JSONiq
Data independence with
heterogeneous, denormalized data.
JSONiq Data Model (JDM): Sequences of Items;
Declarative languages,Functional languages and Set-based languages
Declarative languages focus on describing what the program should accomplish, rather than specifying how to achieve it. Functional languages treat computation as the evaluation of mathematical functions and avoid changing state or mutable data.Haskell, Lisp, and Erlang are functional programming languages.
Parts of JavaScript and Python support functional programming paradigms. Set-based languages are a subset of declarative languages that focus on manipulating sets of data.
It’s worth noting that languages can often belong to more than one category. For example, SQL is both declarative and set-based, and functional programming concepts can be integrated into languages that are not purely functional.
FLWOR clauses
query,Abstract Syntax Tree, Expression Tree, Iterator Tree
Materialized execution,Streamed execution,Parallel execution
Materialized execution takes lots of space. Streamed execution takes lots of time.
Parallel execution can take lots of machines.
Execution modes determined statically for every expression and clause.
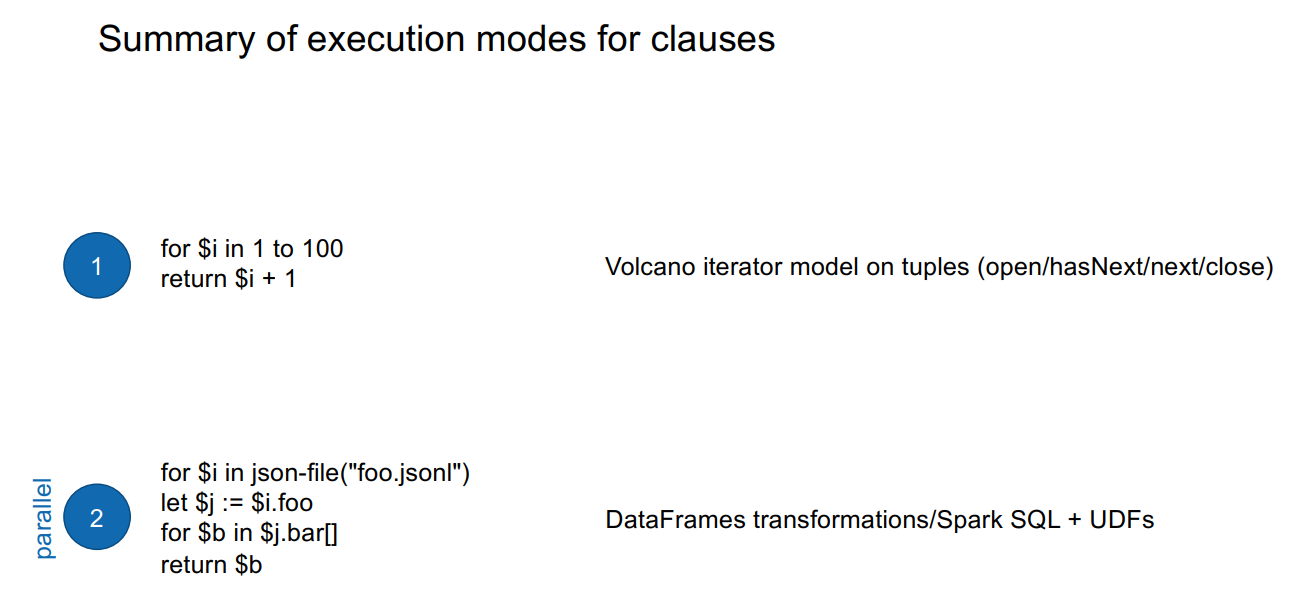
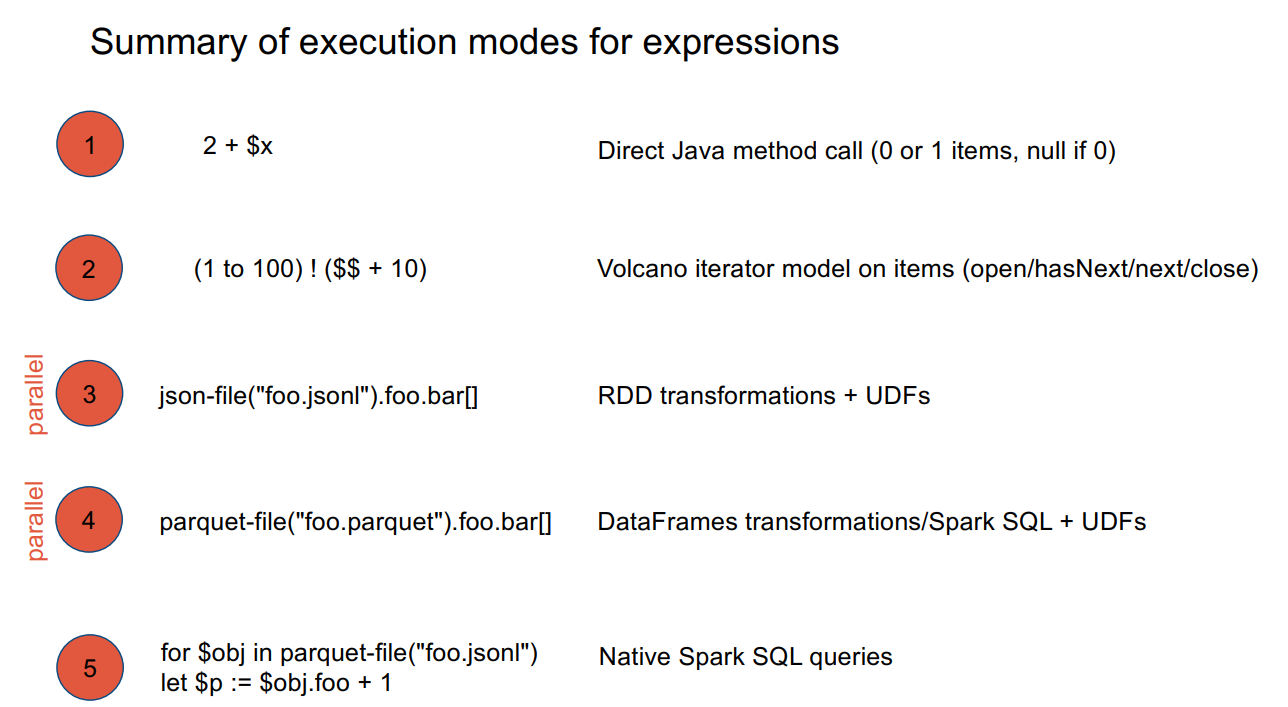
Rumble
Traditional RDBMS/warehouses vs. data lakes
book
exercise
Document Stores
slides
JSON and XML
SQL and NoSQL:
NoSQL: validation after the data was populated.
CRUD
Create,Read,Update,Delete
MongoDB
projecting away: not selecting a field.
hash indices,Tree indices (B+-tree),compund index
Limitations of hash indices:
No support for range queries,Hash function not perfect in real life, Space requirements for collision avoidance.
B+-tree: All leaves at same depth, All non-leaf nodes have between 3 and 5 children,But it’s fine if the root has less.
book
exercise
Performance at Large Scales
slides
sources of bottleneck
Memory, CPU, Disk I/O, Network I/O.
Sweet Spot for MapReduce and Spark: Disk I/O.
Latency, Throughput,Response time
Latency: When do I start receiving data.
Throughput:”How fast can we transmit data.
Response time=Latency + Transfer.
speedup,Amdahl’s law,Gustafson’s law
speedup = latency(old)/latency(new).
Gustafson’s law:Constant computing power.
Amdahl’s law: Constant problem size.
Scaling out,Scaling up
tail latency, SLA
book
exercise
Massive Parallel Processing I(MapReduce)
slides
combine and reduce
map task and reduce task, map slot and reduce slot, map phase amd reduce phase
no combine task and combine slot.
map slot = sequential map task; map task = sequential split map;
split(mapreduce) and block(HDFS)
1 split = 1 map task
split(mapreduce)!= block(HDFS)
book
mapreduce model
In MapReduce, the input data, intermediate data, and output data are all made of a large collection of key-value pairs (with the keys not necessarily unique, and not necessarily sorted by key).
MapReduce architecture
In the original version of MapReduce, the main node is called JobTracker, and the worker nodes are called TaskTrackers.
In fact, the JobTracker typically runs on the same machine as the NameNode (and HMaster) and the TaskTrackers on the same machines as the DataNodes (and RegionServers). This is called “bring the query to the data.”
Note that shuffling can start before the map phase is over, but the reduce phase can only start after the map phase is over.
combine
Combining happens during the map phase.
In fact, in most of the cases, the combine function will be identical to the reduce function, which is generally possible if the intermediate key-value pairs have the same type as the output key-value pairs, and the reduce function is both associative and commutative.
MapReduce programming API
In Java, the user needs to define a so-called Mapper class that contains the map function, and a Reducer class that contains the reduce function.
A map function takes in particular a key and a value. Note that it outputs key-value pairs via the call of the write method on the context, rather than with a return statement.
A reduce function takes in particular a key and a list of values.
function,task,slot,phase
A map function is a mathematical, or programmed, function that takes one input key-value pair and returns zero, one or more intermediate key-value pairs.
Then, a map task is an assignment (or “homework”, or “TODO”) that consists in a (sequential) series of calls of the map function on a subset of the input.
There is no such thing as a combine task. Calls of the combine function are not planned as a task, but is called ad-hoc during flushing and compaction.
The map tasks are processed thanks to compute and memory resources (CPU and RAM). These resources are called map slots. One map slot corresponds to one CPU core and some allocated memory. Each map slot then processes one map task at a time, sequentially.
The map phase thus consists of several map slots processing map tasks in parallel.
short-circuiting(split and block)
This is because the DataNode process of HDFS and the TaskTracker process of MapReduce are on the same machine. Thus, getting a replica of the block containing the data necessary to the processing of the task is as simple as a local read. This is called short-circuiting.
split(logical level)!=HDFS block(physical level).
exercise
Resource management
book
YARN
YARN means Yet Another Resource manager. It was introduced as an additional layer that specifically handles the management of CPU and memory resources in the cluster.
YARN, unsurprisingly, is based on a centralized architecture in which the coordinator node is called the ResourceManager, and the worker nodes are called NodeManagers. NodeManagers furthermore provide slots (equipped with exclusively allocated CPU and memory) known as containers.
YARN provides generic support for allocating resources to any application and is application-agnostic. When the user launches a new application, the ResourceManager assigns one of the container to act as the ApplicationMaster which will take care of running the application.
Version 2 of MapReduce works on top of YARN by leaving the job lifecycle management to an ApplicationMaster.
It is important to understand that, unlike the JobTracker, the ResourceManager does not monitor tasks, and will not restart slots upon failure. This job is left to the ApplicationMasters.
Scheduling strategies
FIFO scheduling,Capacity scheduling,Fair scheduling
Dominant Resource Fairness algorithm.
The two (or more) dimensions are projected again to a single dimension by looking at the dominant resource for each user.
Massive Parallel Processing II (Spark)
slides
YARN
ResourceManager + NodeManager; ResourceManager allocates one NodeManager as application master. Application Master communicates with containers. ResourceManager
Does not monitor tasks and Does not restart upon failure. Fault tolerance is on the application master.
spark
Full-DAG query processing.distributed acyclic graph (DAG)
RDD: Resilient Distributed Dataset.
RDD
lazy evaluation:Lazy evaluation means that the execution of transformations on RDDs is deferred until an action is triggered. Instead of immediately executing the transformations, Spark keeps track of the sequence of transformations in the form of a logical execution plan. The actual computation is only performed when an action is called.
A narrow dependency (also known as a narrow transformation) occurs when each partition of the parent RDD contributes to at most one partition of the child RDD. In other words, the number of partitions remains the same before and after the transformation, and each partition of the child RDD depends on a one-to-one relationship with partitions of the parent RDD.
A wide dependency (also known as a wide transformation) occurs when each partition of the parent RDD contributes to multiple partitions of the child RDD. This typically involves operations that require data shuffling or redistribution, such as groupByKey or reduceByKey.
Data Frames
book
Resilient distributed datasets(RDDs)
Resilient means that they remain in memory or on disk on a “best effort” basis, and can be recomputed if need be. Distributed means that, just like the collections of key-value pairs in MapReduce, they are partitioned and spread over multiple machines.
A major difference with MapReduce, though, is that RDDs need not be collections of pairs. Since a key-value pair is a particular example of possible value, RDDs are a generalization of the MapReduce model for input, intermediate input and output.
The RDD lifecycle
Creation,Transformation(Mapping or reducing, in this model, become two very specific cases of transformations.),Action.
transformations:
unary transformations: The filter transformation,The map transformation,The flatMap transformation,The distinct transformation,The sample transformation
Binary transformations: taking the union of two RDDs, take the intersection, take the subtraction.
Pair transformations: Spark has transformations specifically tailored for RDDs of key-value pairs: The key transformation,The values transformation,The reduceByKey transformation, The groupByKey transformation,The sortByKey transformation,The mapValues transformation,The join transformation,The subtractByKey transformation.
Actions: The collect action,The count action,The countByValue action,The take action,The top action,The takeSample action,The reduce action,saveAsTextFile action,saveAsObjectFile action.
Note that Spark, at least in its RDD API, is not aware of any particular format or syntax, i.e., it is up to the user to parse and serialize values to the appropriate text or bytes.
Actions on Pair RDDs: There are actions specifically working on RDDs of key-value pairs:The countByKey action, The lookup action,
Lazy evaluation
It is only with an action that the entire computation pipeline is put into motion, leading to the computation of all the necessary intermediate RDDs all the way down to the final output corresponding to the action.
Physical architecture
There are two kinds of transformations: narrow-dependency transformations and wide-dependency transformations.
Such a chain of narrow-dependency transformations executed efficiently as a single set of tasks is called a stage, which would correspond to what is called a phase in MapReduce.
Optimizations
Pinning RDDs, Pre-partitioning.
DataFrames in Spark
A DataFrame can be seen as a specific kind of RDD: it is an RDD of rows (equivalently: tuples, records) that has relational integrity, domain integrity, but not necessarily (as the name “Row” would otherwise fallaciously suggest) atomic integrity.
Note that Spark automatically infers the schema from discovering the JSON Lines file, which adds a static performance overhead that does not exist for raw RDDs: there is no free lunch.
Unlike the RDD transformation API, there is no guarantee that the execution will happen as written, as the optimizer is free to reorganize the actual computations.
Spark SQL
both GROUP BY and ORDER BY will trigger a shuffle in the system. The SORT BY clause can sort rows within each partition, but not across partitions, i.e., does not induce any shuffling. The DISTRIBUTE BY clause forces a repartition by putting all rows with the same value (for the specified field(s)) into the same new partition.
use both SORT and DISTRIBUTE = the use of another clause, CLUSTER BY.
A word of warning must be given on SORT, DISTRIBUTE and CLUSTER clauses: they are, in fact, a breach of data independence, because they expose partitions.
explode() and lateral view: Lateral views are more powerful and generic than just an explode() because they give more control, and they can also be used to go down several levels of nesting.
book
exercise
Spark’s RDDs are by default recomputed each time you run an action on them. Please note that both persist() and cache() are lazy operations themselves. The caching operation will, in fact, only take place when the first action is called. With successive action calls, the cached RDD will be used.
introduction
book
three Vs: Volume, Variety, Velocity.
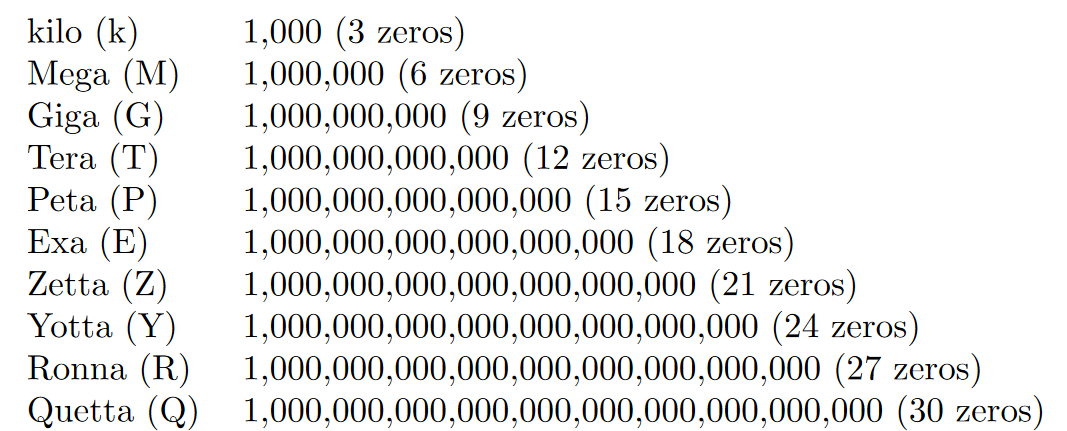
four more shapes: trees, unstructured, cubes,graphs.
three factors: Capacity,Throughput, Latency.
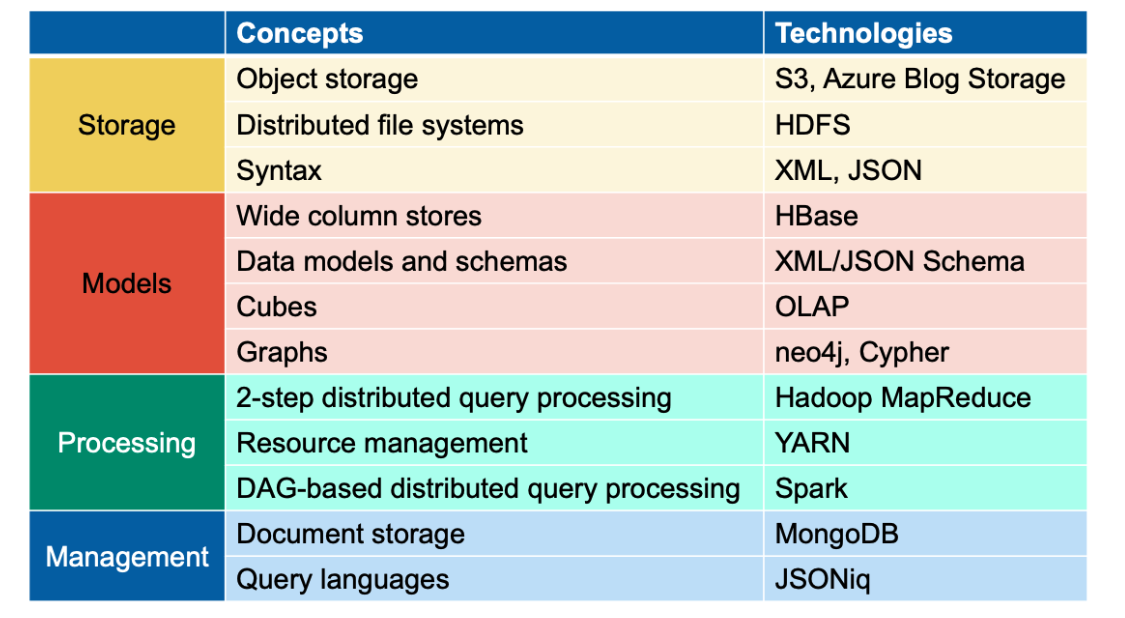
partial function and function
A function is a strict mapping where every element in the domain is mapped to a unique element in the codomain.
A partial function is a mapping where not every element in the domain necessarily has a defined value in the codomain.
For a table, we need to throw in three additional constraints: relational integrity, domain integrity and atomic integrity.
lessons learned from the past
book
natural join, theta join, and outer join,Semi-outer join
A natural join is a type of join that combines rows from two tables based on columns with the same name and data type. The columns used for the join condition are not explicitly specified; instead, the database system automatically identifies the matching columns. A theta join is a generalization of the natural join, where the join condition is explicitly specified using a comparison operator.
Normal forms
The first normal form was already covered earlier: it is in fact atomic integrity.
The second normal form takes it to the next level: it requires that each column in a record contains information on the entire record. The third normal form additionally forbids functional dependencies on anything else than the primary key.
SQL
SQL is a declarative language, which means that the user specifies what they want, and not how to compute it: it is up to the underlying system to figure out how to best execute the query. It is also a set-based language, in the sense that it manipulates sets of records at a time, rather than single values as is common in other languages. It is also, to some limited extent, a functional language in the sense that it contains expressions that can nest in each other (nested queries).
ACID
There are four main properties (often called ACID):Atomicity,Consistency,Isolation,Durability.
exercise
SQL
1NF,2NF,3NF,BCNF;
intersection;
Storing data
book
CAP
(Atomic) Consistency, Availability, Partition tolerance.
document stores
book
Document stores provide a native database management system for semi-structured data. A document store typically specializes in either JSON or XML data, even though some companies (e.g., MarkLogic) offer support for both. It is important to understand that document stores are optimized for the typical use cases of many records of small to medium sizes. Typically, a collection can have millions or billions of documents, while each single document weighs no more than 16 MB (or a size in a similar magnitude).
MongoDB
In MongoDB, the format is a binary version of JSON called BSON.
The API of MongoDB, like many document stores, is based on the CRUD paradigm. CRUD means Create, Read, Update, Delete.
MongoDB automatically adds to every inserted document a special field called “ id” and associated with a value called an Object ID and with a type of its own.
hash indices and tree indices
Hash indices are used to optimize point queries and more generally query that select on a specific value of a field.
Secondary indices
By default, MongoDB always builds a tree index for the id field. Users can request to build hash and tree indices for more fields. These indices are called secondary indices.
exercise
By default, MongoDB creates the _id index, which is an ascending unique index on the _id field, for all collections when the collection is created. You cannot remove the index on the _id field.
Querying denormalized data
book
Features of a query language
First, it is declarative. This means that the users do not focus on how the query is computed, but on what it should return.
Second, it is functional. This means that the query language is made of composable expressions that nest with each other, like a Lego game.
Finally, it is set-based, in the sense that the values taken and returned by expressions are not only single values (scalars), but are large sequences of items (in the case of SQL, an item is a row).
JSONiq
It is possible to filter any sequence with a predicate, where .c = 3]”
To access the n-th member of an array, you can use double-squarebrackets: “json-doc(“file.json”).o[[2]].a”.
Do not confuse sequence positions (single square brackets) with array positions (double square brackets)!
The empty sequence enjoys special treatment: if one of the sides (or both) is the empty sequence, then the arithmetic expression returns an empty sequence (no error).
Note that unlike SQL, JSONiq logic expressions are two-valued and return either true or false.
general comparison
universal and existential quantification: every and some;
JSONiq has a shortcut for existential quantification on value comparisons. This is called general comparison.
FLWOR expressions
One of the most important and powerful features of JSONiq is the FLWOR expression. It corresponds to SELECT-FROM-WHERE queries in SQL.
exercise
Accessing a JSON dataset can be done in two ways depending on the exact format:
If this is a file that contains a single JSON object spread over multiple lines, use json-doc(URL).
If this is a file that contains one JSON object per line (JSON Lines), use json-file(URL).
HDFS
book
HDFS data model
HDFS does not follow a key-value model: instead, an HDFS cluster organizes its files as a hierarchy, called the file namespace. Files are thus organized in directories, similar to a local file system.
key-value model vs file hierarchy
The key-value model and file hierarchy are two different approaches to organizing and accessing data within storage systems. While the key-value model excels in flexibility and quick data access, file hierarchy provides a structured and predictable organization suitable for many traditional storage use cases.
object storage vs block storage
Organizes data as objects, each containing both data and metadata. Objects are stored in a flat address space.
Organizes data as fixed-size blocks, typically within storage volumes.Requires a file system to manage and organize data into files and directories.
object storage and key-value model
Object storage is a data storage architecture that manages data as objects, each containing both data and metadata. Objects are stored in a flat address space without the hierarchy found in traditional file systems. In the key-value model, data is organized as pairs of keys and values. Each key uniquely identifies a value, and the system allows for the efficient retrieval and storage of data based on these key-value pairs.
Object storage systems often use a key-value model internally to manage objects. Each object has a unique identifier (key), and the associated data and metadata form the corresponding value.
physical architecture
In the case of HDFS, the central node is called the NameNode and the other nodes are called the DataNodes. In fact, more precisely, the NameNode and DataNodes are processes running on these nodes.
The NameNode stores in particular three things:the file namespace,a mapping from each file to the list of its blocks, a mapping from each block, represented with its 64-bit identifier, to the locations of its replicas.
exercise
object storage vs block storage
syntax
book
json
JSON stands for JavaScript Object Notation.
JSON is made of exactly six building blocks: strings, numbers, Booleans, null, objects, and arrays.
in JSON, escaping is done with backslash characters (\).
The way a number appears in syntax is called a lexical representation, or a literal.
JSON places a few restrictions: a leading + is not allowed. Also, a leading 0 is not allowed except if the integer part is exactly 0 (in which case it is even mandatory).
Objects are simply maps from strings to values. The keys of an object must be strings.
The JSON standard recommends for keys to be unique within an object.
unicode
Unicode is a standard that assigns a numeric code (called a code point) to each character in order to catalogue them across all languages of the world, even including emojis. The code point must be indicated in base 16.
XML
XML stands for eXtensible Markup Language.
XML’s most important building blocks are elements, attributes, text and comments.
Unlike JSON keys, element names can repeat at will.
At the top-level, a well-formed XML document must have exactly one element.
Attributes appear in any opening elements tag and are basically keyvalue pairs. Values can be either double-quoted or single-quoted. The key is never quoted, and it is not allowed to have unquoted values. Within the same opening tag, there cannot be duplicate keys. Attributes can never appear in a closing tag. It is not allowed to create attributes that start with XML or xml, or any case combination. because this is reserved for namespace declarations.
a single comment alone is not well-formed XML (remember: we need exactly one top-level element).
XML documents can be identified as such with an optional text declaration containing a version number and an encoding.
Another tag that might appear right below, or instead of, the text declaration is the doctype declaration. It must then repeat the name of the top-level element.
Remember that in JSON, it is possible to escape sequences with a backslash character. In XML, this is done with an ampersand (&) character.
Escape sequences can be used anywhere in text, and in attribute values.there are a few places where they are mandatory: In text, & and < MUST be escaped. In double-quoted attribute values, ”, & and < MUST be escaped. In single-quoted attribute values, ’, & and < MUST be escaped.
Namespaces in XML
A namespace is identified with a URI.
The triplet (namespace, prefix, localname) is called a QName (for “qualified name”).
For the purpose of the comparisons of two QNames (and thus of documents), the prefix is ignored: only the local name and the namespace are compared.
First, unprefixed attributes are not sensitive to default namespaces: unlike elements, the namespace of an unprefixed attribute is always absent even if there is a default namespace.
exercise
xml names
Remember:
Element names are case-sensitive.
Element names must start with a letter or underscore.
Element names cannot start with the letters xml (or XML, or Xml, etc).
Element names can contain letters, digits, hyphens, underscores, and periods.
Element names cannot contain spaces.
JSON Key names
The only restriction the JSON syntax imposes on the key names is that “ and \ must be escaped.
Wide column stores
book
HDFS VS Wide column stores
The problem with HDFS is its latency: HDFS works well with very large files (at least hundreds of MBs so that blocks even start becoming useful), but will have performance issues if accessing millions of small XML or JSON files. Wide column stores were invented to provide more control over performance and in particular, in order to achieve high-throughput and low latency for objects ranging from a few bytes to about 10 MB, which are too big and numerous to be efficiently stored as so-called clobs (character large objects) or blobs (binary large objects) in a relational database system, but also too small and numerous to be efficiently accessed in a distributed file system.
object storage VS Wide column stores
a wide column store has additional benefits: a wide column store will be more tightly integrated with the parallel data processing systems.wide column stores have a richer logical model than the simple key-value model behind object storage; wide column stores also handle very small values (bytes and kBs) well thanks to batch processing.
HBase
From an abstract perspective, HBase can be seen as an enhanced keyvalue store, in the sense that:a key is compound and involves a row, a column and a version; keys are sortable; values can be larger (clobs, blobs), up to around 10 MB. On the logical level, the data is organized in a tabular fashion: as a collection of rows. Each row is identified with a row ID. Row IDs can be compared, and the rows are logically sorted by row ID. Column qualifiers are arrays of bytes (rather than strings), and as for row IDs, there is a library to easily create column qualifiers from primitive values.
HBase supports four kinds of low-level queries: get, put, scan and delete. Unlike a traditional key-value store, HBase also supports querying ranges of row IDs and ranges of timestamps.
HBase offers a locking mechanism at the row level, meaning that different rows can be modified concurrently, but the cells in the same row cannot: only one user at a time can modify any given row.
A table in HBase is physically partitioned in two ways: on the rows and on the columns. The rows are split in consecutive regions. Each region is identified by a lower and an upper row key, the lower row key being included and the upper row key excluded. A partition is called a store and corresponds to the intersection of a region and of a column family.
All the cells within a store are eventually persisted on HDFS, in files that we will call HFiles. An HFile is, in fact, nothing else than a (boring) flat list of KeyValues, one per cell. What is important is that, in an HFile, all these KeyValues are sorted by key in increasing order.
HDFS block and HBlock
HBase uses index structures to quickly skip to the position of the HBase block which may hold the requested key. Note HBase block is not to be confused with HDFS block and the underlying file system block.By default, each HBase block is 64KB (configurable) in size and always contains whole key-value pairs, so, if a block needs more than 64KB to avoid splitting a key-value pair, it will just grow.
Log-structured merge trees
Upon flushing, all cells are written sequentially to a new HFile in ascending key order, HBlock by HBlock, concurrently building the index structure. In fact, sorting is not done in the last minute when flushing. Rather, what happens is that when cells are added to memory, they are added inside a data structure that maintains them in sorted order (such as tree maps) and then flushing is a linear traversal of the tree.
Bloom filters
HBase has a mechanism to avoid looking for cells in every HFile. This mechanism is called a Bloom filter. It is basically a black box that can tell with absolute certainty that a certain key does not belong to an HFile, while it only predicts with good probability (albeit not certain) that it does belong to it.
exercise
Bloom filters
Bloom filters are a data structure used to speed up queries, useful in the case in which it’s likely that the value we are looking doesn’t exist in the collection we are querying. Their main component is a bit array with all values initially set to 0. When a new element is inserted in the collection, its value is first run through a certain number of (fixed) hash functions, and the locations in the bit array corresponding to the outputs of these functions are set to 1.
This means that when we query for a certain value, if the value has previously been inserted in the collection then all the locations corresponding to the hash function outputs will certainly already have been set to 1. On the contrary, if the element hasn’t been previously inserted, then the locations may or may not have already been set to 1 by other elements. Then, if prior to accessing the collection we run our queried value through the hash functions, check the locations corresponding to the outputs, and find any of them to be 0, we are guaranteed that the element is not present in the collection (No False Negatives), and we don’t have to waste time looking. If the corresponding locations are all set to 1, the element may or may not be present in the collection (possibility of False Positives), but in the worst case we’re just wasting time.
As you have seen in the task above, HBase has to check all HFiles, along with the MemStore, when looking for a particular key. As an optimisation, Bloom filters are used to avoid checking an HFile if possible. Before looking inside a particular HFile, HBase first checks the requested key against the Bloom filter associated with that HFile. If it says that the key does not exist, the file is not read.
a Bloom filter can produce false positive outcomes. Luckily, it never produces false negative outcomes.
Log-structured merge-tree (LSM tree) (optional)
As opposed to B+-tree which has a time complexity of O(log n) when inserting new elements, n being the total number of elements in the tree, LSM tree has O(1) for inserting, which is a constant cost.
Data models and validation
book
A data model is an abstract view over the data that hides the way it is stored physically. For example, a CSV file should be abstracted logically as a table.
The JSON Information Set
it is possible to take a tree and output it back to JSON syntax. This is called serialization.
The XML Information Set
A fundamental difference between JSON trees and XML trees is that for JSON, the labels (object keys) are on the edges connecting an object information item to each one of its children information items. In XML, the labels (these would be element and attribute names) are on the nodes (information items) directly.
Item types
Also, all atomic types have in common that they have a logical value space and a lexical value space. Atomic types can be in a subtype relationship: a type is a subtype of another type if its logical value space is a subset of the latter.
However, in modern databases, it is customary to support unbounded integers.
Decimals correspond to real numbers that can be written as a finite sequence of digits in base 10, with an optional decimal period.Support for the entire decimal value space can be costly in performance. In order to address this issue, a floating-point standard (IEEE 754) was invented and is still very popular today.
Timestamp values are typically stored as longs (64-bit integers) expressing the number of milliseconds elapsed since January 1, 1970 by convention.
XML Schema, JSound and JSONiq follow the ISO 8601 standard.
The lexical representation of duration can vary, but there is a standard defined by ISO 8601 as well, starting with a P and prefixing sub-day parts with a T.
Maps (not be confused with records, which are similar) are maps from any atomic value to any value, i.e., generalize objects to keys that are not necessarily strings (e.g., numbers, dates, etc). However, unlike records, the type of the values must be the same for all keys.
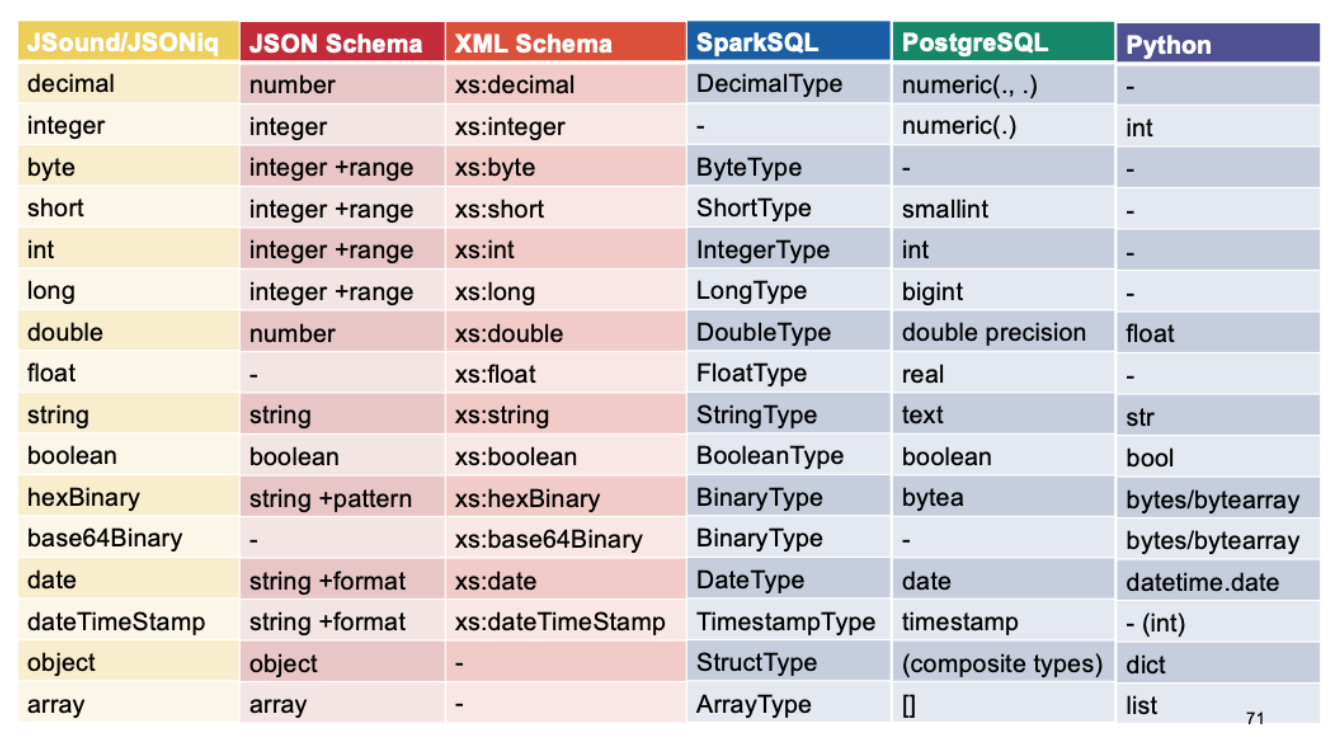
JSound and JSON Schema
JSound is a schema language that was designed to be simple for 80% of the cases, making it particularly suitable in a teaching environment. It is independent of any programming language.
JSON Schema is another technology for validating JSON documents.
The type system of JSON Schema is thus less rich than that of JSound, but extra checks can be done with so-called formats, which include date, time, duration, email, and so on including generic regular expressions.
It is possible to require the presence of a key by adding an exclamation mark in JSound. in JSON Schema, which uses a “required” property associated with the list of required keys to express the same.
In the JSound compact syntax, extra keys are forbidden. Unlike JSound, in JSON Schema, extra properties are allowed by default. JSON Schema then allows to forbid extra properties with the “additionalProperties” property.
There are a few more features available in the compact JSound syntax (not in JSON Schema) with the special characters @, ? and =. The question mark (?) allows for null values (which are not the same as absent values). The arobase (@) indicates that one or more fields are primary keys for a list of objects that are members of the same array. The equal sign (=) is used to indicate a default value that is automatically populated if the value is absent.
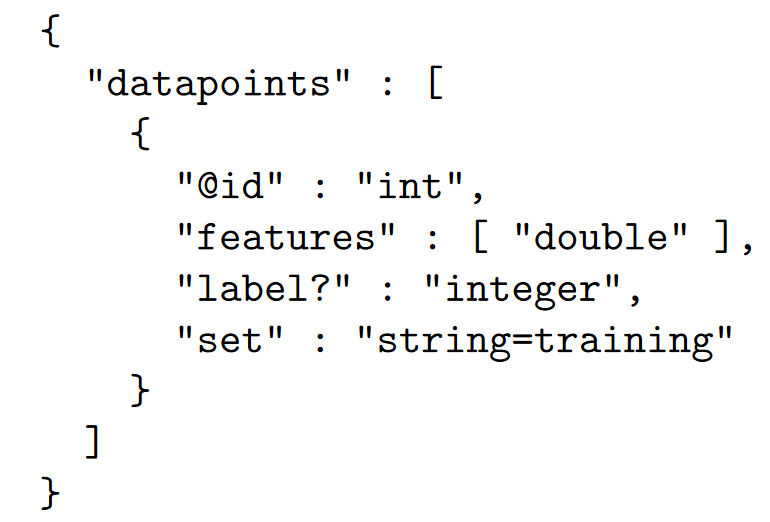
Note that some values are quoted, which does not matter for validation: validation only checks whether lexical values are part of the type’s lexical space.
Accepting any values in JSound can be done with the type “item”, which contains all possible values. In JSON Schema, in order to declare a field to accept any values, you can use either true or an empty object in lieu of the type.
JSON Schema additionally allows to use false to forbid a field.
In JSON Schema, it is also possible to combine validation checks with Boolean combinations using “anyOf”.JSound schema allows defining unions of types with the vertical bar inside type strings.
In JSON Schema only (not in JSound), it is also possible to do a conjunction (logical and) with “allOf” as well as exclusive with “oneOf” as well as negation with “not”.
XML Schema
all elements in an XML Schema are in a namespace, the XML Schema namespace. The namespace is prescribed by the XML Schema standard and must be this one.
dataframe
There is a particular subclass of semi-structured datasets that are very interesting: valid datasets, which are collections of JSON objects valid against a common schema, with some requirements on the considered schemas. The datasets belonging to this particular subclass are called data frames, or dataframes.
relational tables are data frames, while data frames are not necessarily relational tables: data frames can be (and are often) nested, but they are still relatively homogeneous to some extent.Thus, Data frames are a generalization of (normalized) relational tables allowing for (organized and structured) nestedness.
data format
In fact, if the data is structured as a (valid) data frame, then there are many, many different formats that it can be stored in, and in a way that is much more efficient than JSON. These formats are highly optimized and typically stored in binary form, for example Parquet, Avro, Root, Google’s protocol buffers, etc.
Why is it possible to store the data more efficiently when it is valid and data-frame-friendly? One important reason is that the schema can be stored as a header in the binary format, and the data can be stored without repeating the fields in every record (as is done in textual JSON).
exercise
Dremel
Dremel is a query system developed at Google for deriving data stored in a nested data format such as XML, JSON, or Google Protocol Buffers into column storage, where it can be analyzed faster.
paper notes
Dynamo
CAP: AP.
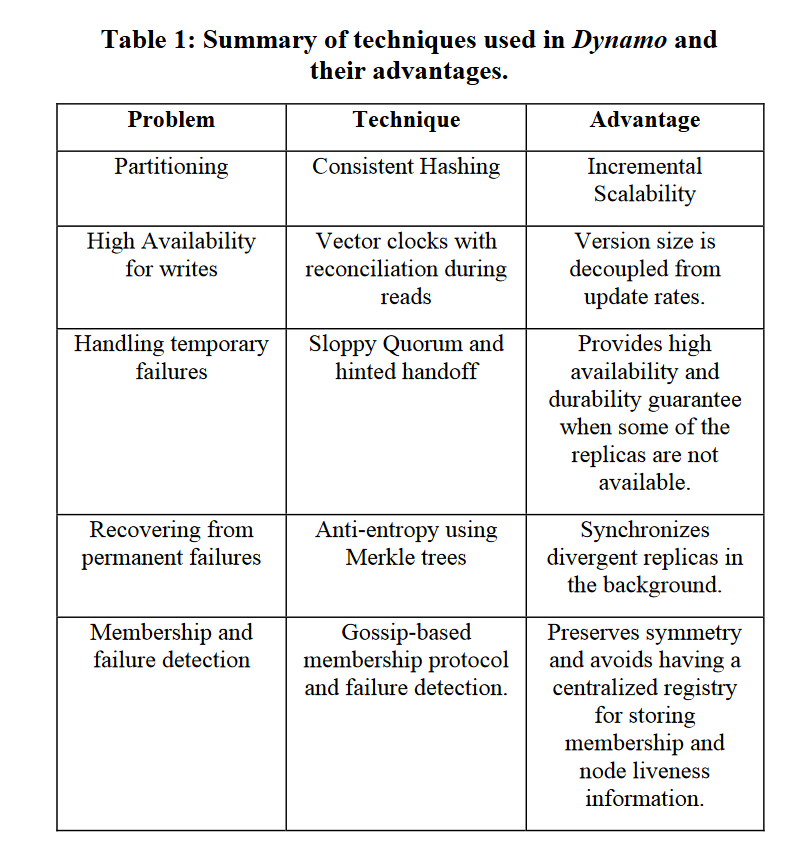
preference list and coordinator
A node handling a read or write operation is known as the coordinator. Typically, this is the first among the top N nodes in the preference list.
quorum-like system
To maintain consistency among its replicas, Dynamo uses a consistency protocol similar to those used in quorum systems. This protocol has two key configurable values: R and W. R is the minimum number of nodes that must participate in a successful read operation. W is the minimum number of nodes that must participate in a successful write operation. Setting R and W such that R + W > N yields a quorum-like system.
To remedy this it does not enforce strict quorum membership and instead it uses a “sloppy quorum”; all read and write operations are performed on the first N healthy nodes from the preference list.
Merkle Trees
A hash tree or Merkle tree is a binary tree in which every leaf node gets as its label a data block and every non-leaf node is labelled with the cryptographic hash of the labels of its child nodes. Some KeyValue stores use Merkle trees for efficiently detecting inconsistencies in data between replicas.
vector clock
Dremel
In contrast to layers such as Pig19 and Hive,16 it executes queries natively without translating them into MR jobs. Lastly, and importantly, Dremel uses a column-striped storage representation, which enables it to read less data from secondary storage and reduce CPU cost due to cheaper compression.
Repetition and definition levels
we define the repetition level as the number of repeated fields in the common prefix (including the first path element identifying the record). The definition level specifies the number of optional and repeated fields in the path (excluding the first path element).A definition level smaller than the maximal number of repeated and optional fields in a path denotes a NULL.
HDFS
Each block replica on a DataNode is represented by two files in the local host’s native file system. The first file contains the data itself and the second file is block’s metadata including checksums for the block data and the block’s generation stamp.
If the NameNode does not receive a heartbeat from a DataNode in ten minutes the NameNode considers the DataNode to be out of service and the block replicas hosted by that DataNode to be unavailable. The NameNode then schedules creation of new replicas of those blocks on other DataNodes. Heartbeats from a DataNode also carry information about total storage capacity, fraction of storage in use, and the number of data transfers currently in progress. These statistics are used for the NameNode’s space allocation and load balancing decisions. The NameNode does not directly call DataNodes. It uses replies to heartbeats to send instructions to the DataNodes.
A recently introduced feature of HDFS is the BackupNode. Like a CheckpointNode, the BackupNode is capable of creating periodic checkpoints, but in addition it maintains an inmemory, up-to-date image of the file system namespace that is always synchronized with the state of the NameNode.
A replica stored on a DataNode may become corrupted because of faults in memory, disk, or network. HDFS generates and stores checksums for each data block of an HDFS file. Checksums are verified by the HDFS client while reading to help detect any corruption caused either by client, DataNodes, or network. When a client creates an HDFS file, it computes the checksum sequence for each block and sends it to a DataNode along with the data. A DataNode stores checksums in a metadata file separate from the block’s data file. When HDFS reads a file, each block’s data and checksums are shipped to the client. The client computes the checksum for the received data and verifies that the newly computed checksums matches the checksums it received. If not, the client notifies the NameNode of the corrupt replica and then fetches a different replica of the block from another DataNode.
The design of HDFS I/O is particularly optimized for batch processing systems, like MapReduce, which require high throughput for sequential reads and writes.
Currently HDFS provides a configurable block placement policy interface so that the users and researchers can experiment and test any policy that’s optimal for their applications.
When a block becomes over replicated, the NameNode chooses a replica to remove. The NameNode will prefer not to reduce the number of racks that host replicas, and secondly prefer to remove a replica from the DataNode with the least amount of available disk space. When a block becomes under-replicated, it is put in the replication priority queue. A background thread periodically scans the head of the replication queue to decide where to place new replicas.
HDFS block placement strategy does not take into account DataNode disk space utilization. This is to avoid placing new—more likely to be referenced—data at a small subset of the DataNodes.
Each DataNode runs a block scanner that periodically scans its block replicas and verifies that stored checksums match the block data. Whenever a read client or a block scanner detects a corrupt block, it notifies the NameNode. The NameNode marks the replica as corrupt, but does not schedule deletion of the replica immediately. Instead, it starts to replicate a good copy of the block. Only when the good replica count reaches the replication factor of the block the corrupt replica is scheduled to be removed. So even if all replicas of a block are corrupt, the policy allows the user to retrieve its data from the corrupt replicas.
A present member of the cluster that becomes excluded is marked for decommissioning. Once a DataNode is marked as decommissioning, it will not be selected as the target of replica placement, but it will continue to serve read requests. The NameNode starts to schedule replication of its blocks to other DataNodes. Once the NameNode detects that all blocks on the decommissioning DataNode are replicated, the node enters the decommissioned state. Then it can be safely removed from the cluster without jeopardizing any data availability.
MapReduce
The master pings every worker periodically.If no response isrecei ved from awork er in acertain amount of time, the master marks the worker as failed.
json
JSON stands for JavaScript Object Notation and was inspired by the object literals of JavaScript.
A JSON value can be an object, array, number, string, true, false, or null.
The JSON syntax does not impose any restrictions on the strings used as names, does not require that name strings be unique, and does not assign any significance to the ordering of name/value pairs.
Numeric values that cannot be represented as sequences of digits (such as Infinity and NaN) are not permitted.
All strings in JSON must be double-quoted.
rumble
a query execution engine for large, heterogeneous, and nested collections of JSON objects built on top of Apache Spark.
xml
XML, unlike HTML, is case-sensitive. is not the same as or .
Every well-formed XML document has exactly one root element.
XML elements can have attributes. An attribute is a name-value pair attached to the element’s start-tag. Names are separated from values by an equals sign and optional whitespace. Values are enclosed in single or double quotation marks.
each element may have no more than one attribute with a given name.
Element and other XML names may contain essentially any alphanumeric character. This includes the standard English letters A through Z and a through z as well as the digits 0 through 9. They may also include these three punctuation characters: _ The underscore ,- The hyphen, . The period.Finally, all names beginning with the string “XML” (in any combination of case) are reserved for standardization in W3C XML-related specifications. XML names may only start with letters, ideograms, or the underscore character. They may not start with a number, hyphen, or period.
exam notes
2022
HTTP command and status code.
Storage type choosing.
HDFS and random access:
For distributed data storage though, and for the use case at hand where we read a large dataset, analyze it, and write back the output as a new dataset, random access is not needed. A distributed file system is designed so that, in cruise mode, its bottleneck will be the data flow (throughput), not the latency. This aspect of the design is directly consistent with a full-scan pattern, rather than with a random access pattern, the latter being strongly latency-bound.
json and comments:In JSON (JavaScript Object Notation), comments are not officially supported.
Hbase and meta table.
if empty json valid against to some schema.
mapreduce split.
mapreduce function: emit.
if reduce function can be used as combine function.
1NF,2NF and 3NF:
A candidate key is a minimal set of attributes that determines the other attributes included in the relation. A non-prime attribute is an attribute that is not part of the candidate key.Informally, the second normal form states that all attributes must depend on the entire candidate key.In other words, non-prime attributes must be functionally dependent on the key(s), but they must not depend on another non-prime attribute. 3NF non-prime attributes depend on “nothing but the key”.
different type comparision in mongodb.
If you have n dimensions, the CUBE operation will generate 2^n combinations.
xml schema:
You have already seen the xs:sequence element, which dictates that the elements it contains must appear in exactly the same order in which they appear within the sequence element.
mongdb atomic operations:
In MongoDB, a write operation is atomic on the level of a single document, even if the operation modifies multiple embedded documents within a single document.
When a single write operation (e.g. db.collection.updateMany()) modifies multiple documents, the modification of each document is atomic, but the operation as a whole is not atomic.
Json schema:
tuple validation.
references
https://vertabelo.com/blog/normalization-1nf-2nf-3nf/